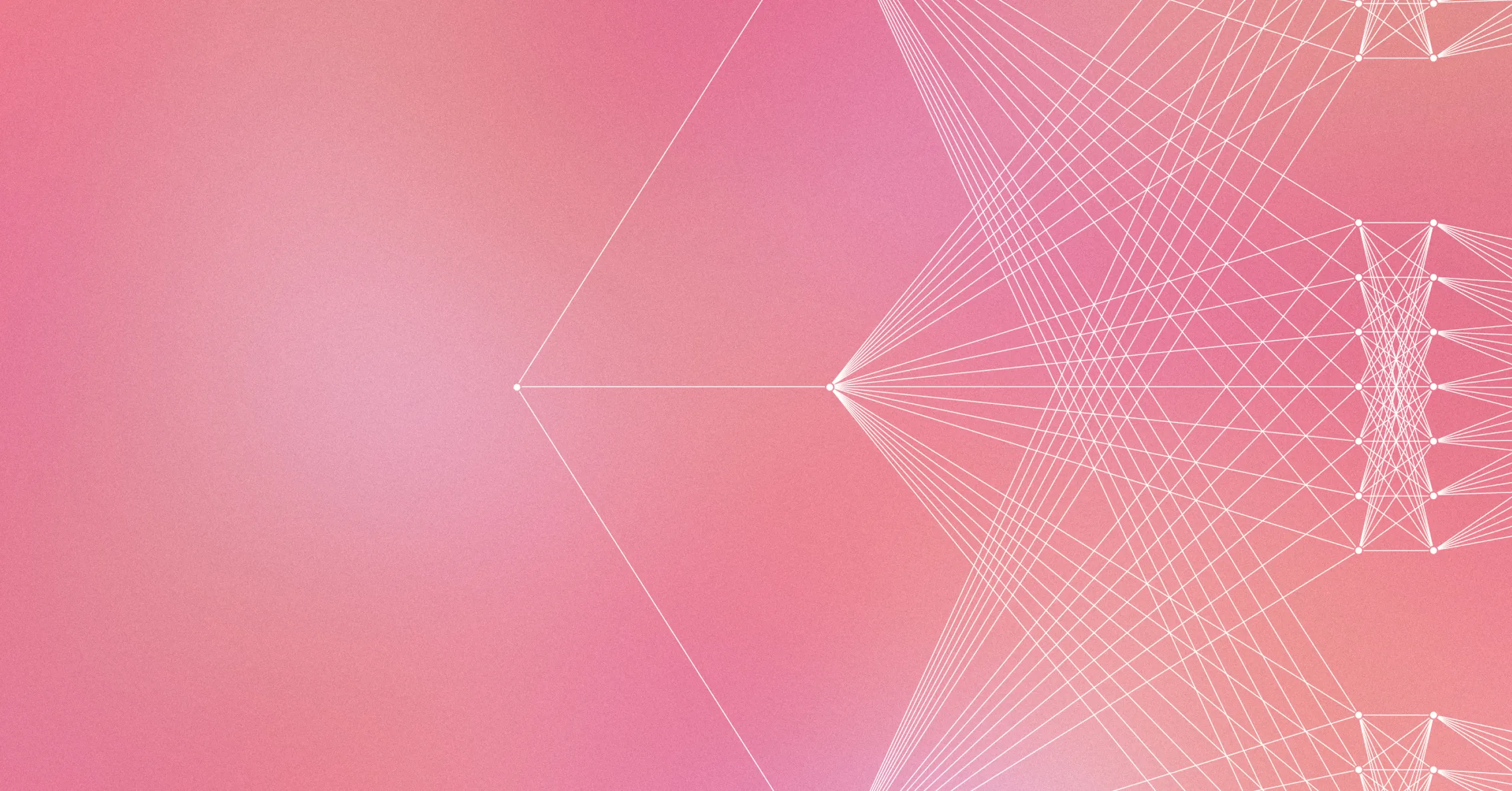
From GenerativeĀ AI to GenerativeĀ UI
The future of AI lies in intelligent interfaces. But what exactly do we mean by this? This report introduces the concept of GenerativeĀ UI ā a fundamental shift in how we create and interact with user interfaces. At its core, GenerativeĀ UI leverages AI modelsā ability to generate code in real time, enabling the creation of entirely new UI elements and interactions on demand.
The rapid progress of GenerativeĀ AI can be attributed to two pivotal innovations. First, GenerativeĀ AIās ability to interpret unstructured data, and second, how the introduction of user-friendly tools like ChatGPT provided a user interface (UI) that enabled users to interact with this data.
By providing AI with a UI, the complexity of Large Language Models (LLMs) was abstracted behind simple, intuitive interfaces. This abstraction allowed users to interact with sophisticated AI systems through natural language or simple prompts, without needing to understand the intricate workings of the underlying models themselves.
So rather than a transformation within AI itself, what rapidly transformed AI from a specialist technology into an everyday tool was to great extent a revolution in UI & UX.
And given the general-purposeness of GenerativeĀ AI, this technology is fast becoming the everyday tool for every industry ā leading to a cascade of innovations and blurring the lines between different technologies and use cases.
However, what began with text summarisation and image creation has evolved into a suite of advanced functionalities spanning video, image, audio, and text. It is clear that chatbots were just the beginning, and multimodal GenAI models like Googleās Gemini are opening the door to a whole new era in human-machine interaction.
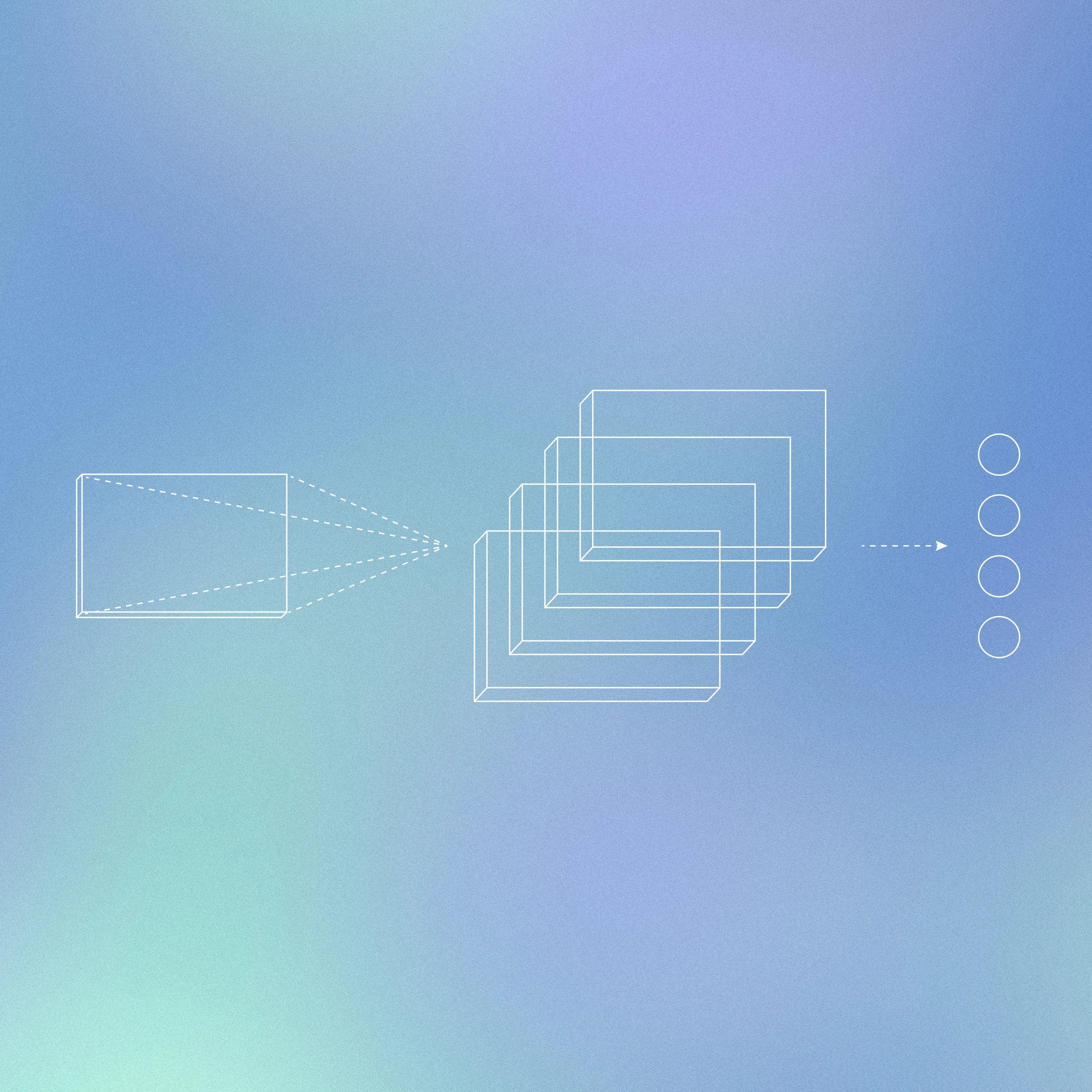
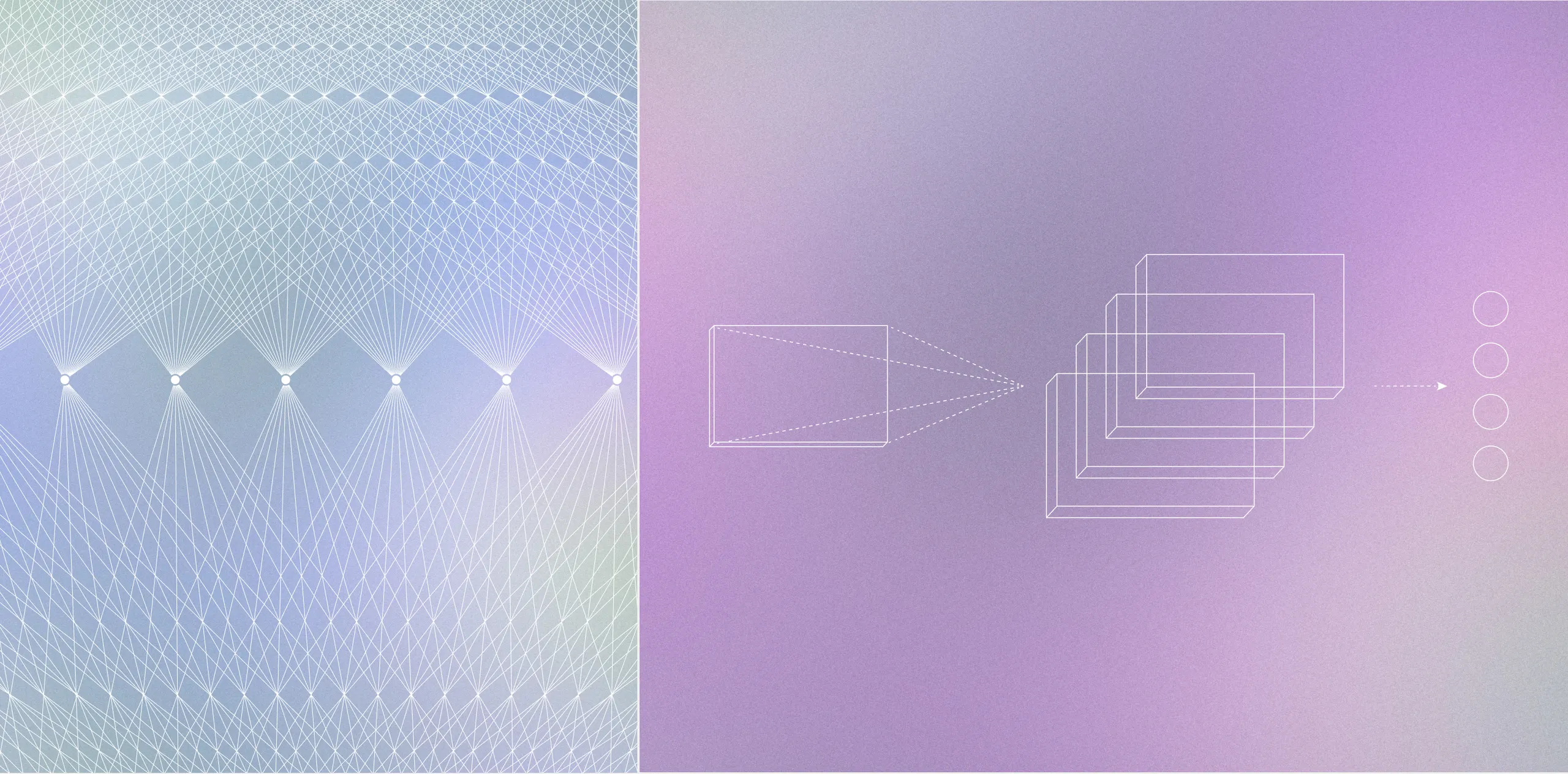
These models can seamlessly integrate information from various sources, enabling more comprehensive analysis, intuitive problem-solving, and innovative content creation across diverse fields. Not only that, but multimodal models enhance AI systems by combining text, image, and audio inputs into a more comprehensive understanding of the data, leading to improved performance across various tasks.
Increasingly, we will see the heavy lifting of interacting with AI move behind the user interface.
As a result, the trajectory remains clearly one in which models are becoming more capable at quickening pace. In the space of just a few years, the ācontext windowsā of Large Language Models have increased exponentially ā now reaching into the millions (for those unaware, context windows refer to the amount of text an AI can consider at once ā essentially its short-term memory). This increased capacity is allowing models to maintain a more comprehensive grasp of information throughout extended interactions.
Not to be caught sleeping, this expansion has ignited a surge of investment and innovation, with £16.83 billion invested globally in 2023 and a further expected £9.48 billion in 2024. At the same time, hardware developments are leading to increasingly potent and efficient computing infrastructures.
The startup Etchedās Sohu chip for instance ā dubbed the worldās first specialised chip for transformers (the āTā in ChatGPT) ā is reported to potentially outperform general-purpose GPUs in both speed and energy efficiency. Similarly, Groq (not to be confused with Grok) has developed what it calls a ālanguage processing unitā (LPU), claiming itās faster and one-tenth the cost of conventional GPUs used in AI.
Elsewhere, developments such as Metaās Llama 3.1 open-weight model mean that this revolution is being democratised. Not only are AI models becoming increasingly multilingual by default, open-sourced models are quickly closing the performance gap on their proprietary counterparts in what is now very much a game of inches. The juryās out on whether or not this will continue as proprietary providers close ranks, but regardless, the adaptation and adoption of more domain-specific models is allowing enterprises to become increasingly AI-enabled in more efficient and practical ways.
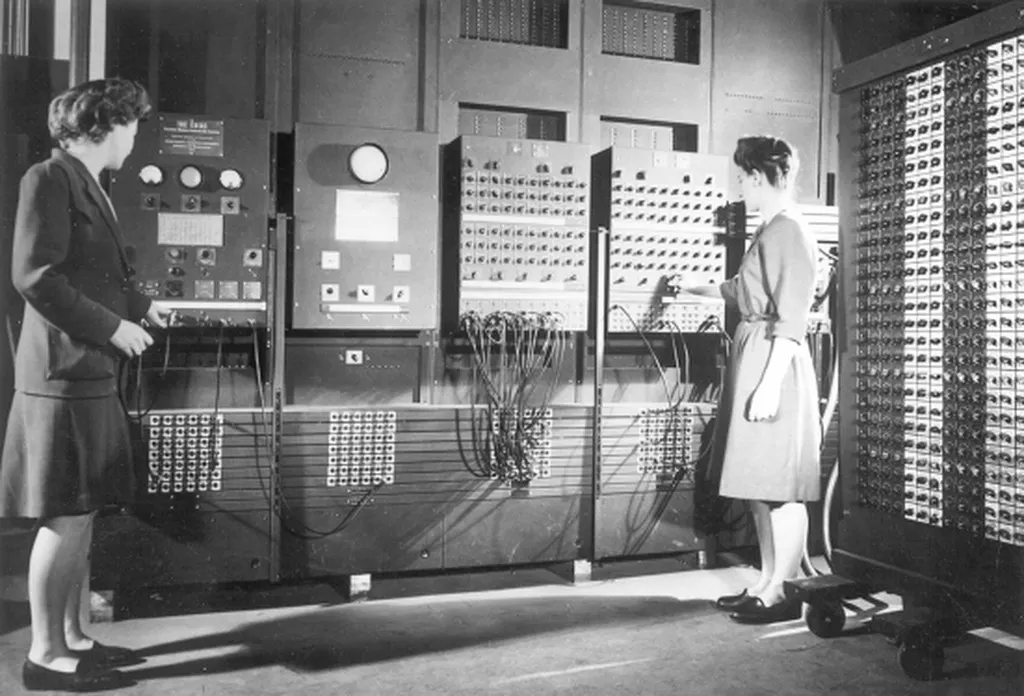
Similarly, we are seeing the arrival of more task-specific Small Language Models (SLMs). Hugging Face, Nvidia (in partnership with Mistral AI), and OpenAI have each released their own SLMs. SLMs are more specialised and require less data and computational power to train, making them ideal for specific tasks or domains. SLMs also enable AI processing at the edge ā addressing critical issues of data privacy and latency by allowing computations to occur on local devices rather than in remote data centres.
The emerging landscape is one where GenerativeĀ AI is no longer a niche technology but a versatile, transformative multi-agent-force reshaping business processes and redefining the boundaries of productivity across industries.
What this means is that, increasingly, we will see the heavy lifting of interacting with AIāspecifically, the complex task of prompting ā move behind the user interface. This shift will allow users to focus on the task at hand, rather than on how they communicate with the AI.
We have seen such developments before. In the early days of computing, users had to know specific commands and syntax to interact with machines, similar to how we currently craft careful prompts for AI systems. The advent of graphical user interfaces (GUIs) made computers accessible to a broader audience, allowing users to focus on their tasks rather than remembering commands. Much like in the past, the future of human-machine interaction lies behind more intelligent interfaces, what we call GenerativeĀ UI.
As this transition occurs, the key to unlocking GenAIās full potential will lie in understanding, designing and developing these intelligent interfaces.
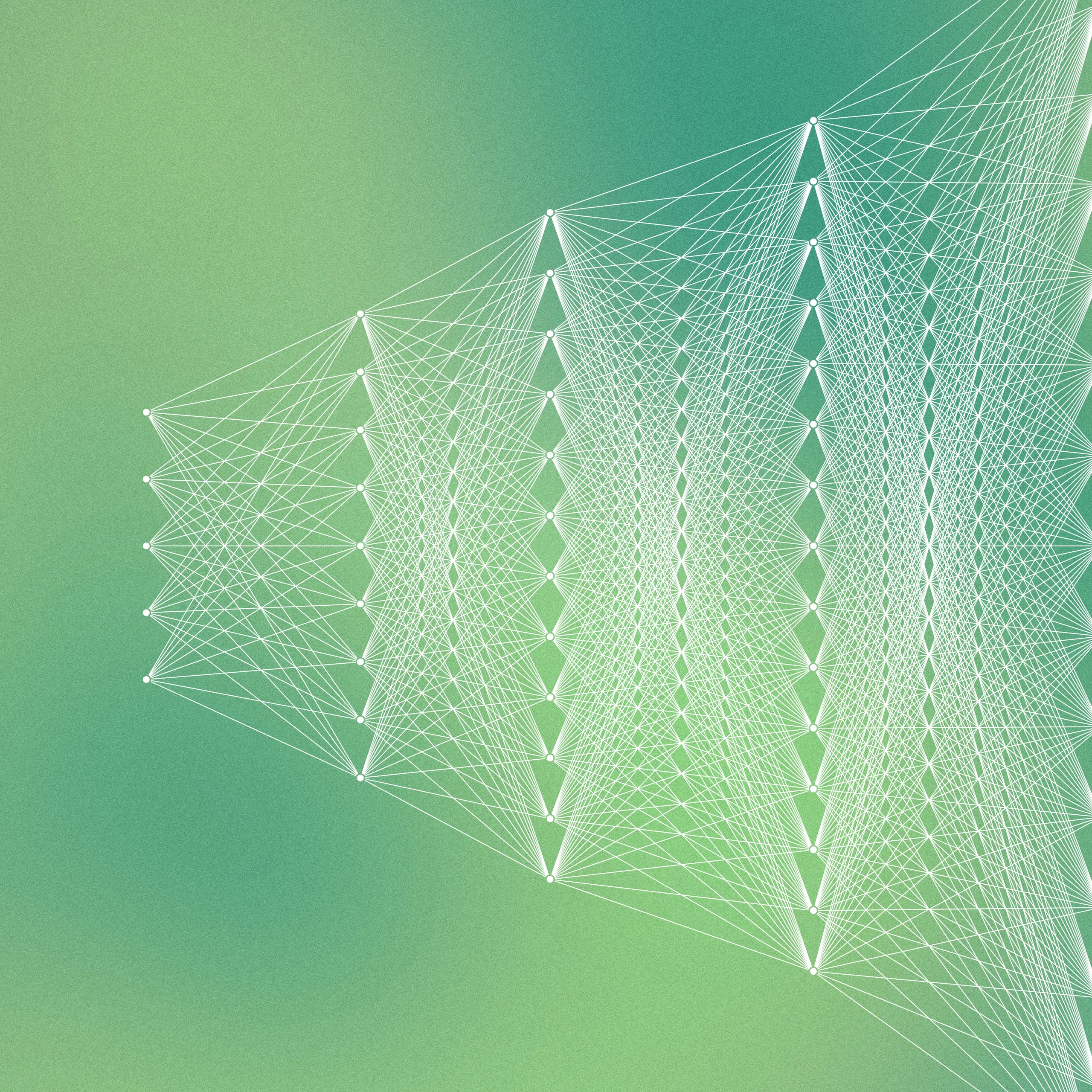
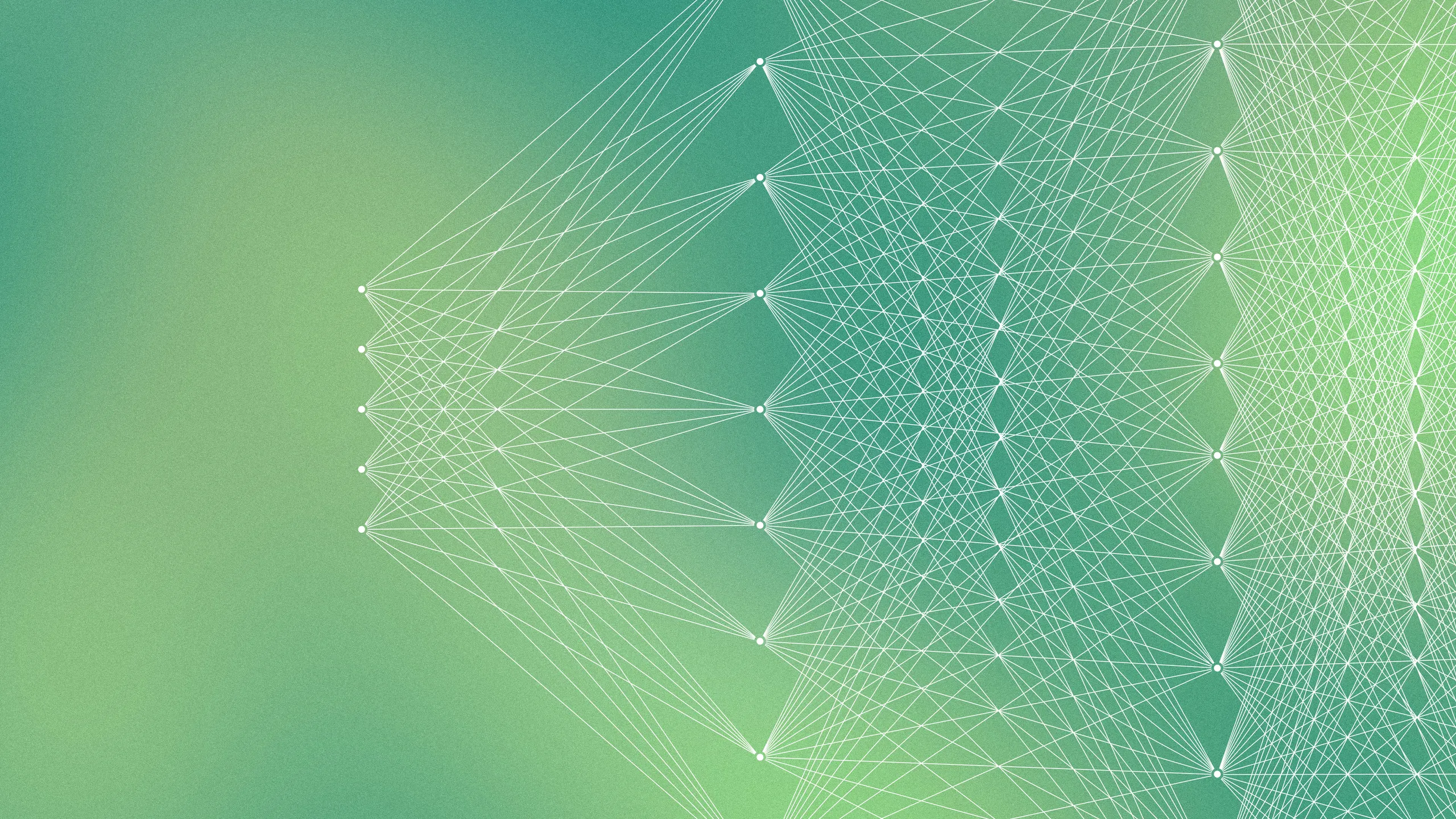
How GenerativeĀ UI is transforming business operations
At a macro level, Generative AI is expected to make big business gains across industries. In the UK alone, a study by Workday predicted AI could create a £119 billion annual productivity boost for large UK enterprises.
At a more granular level, GenerativeĀ AI will supercharge employee productivity, saving a staggering 7.9 billion employee hours annually through the strategic implementation of AI technologies.
Problems in terms of adoption, however, remain. In the same Workplace study, 35% of those surveyed listed inadequate technology, ā legacy apps, outdated solutions or technology thatās a poor-fit for their work ā as holding employees back. Almost the same number (34%) viewed a lack of education as a barrier to utilising AI tools.
But by developing GenerativeĀ AI products that are intuitive and tailored to specific work contexts, GenerativeĀ UI addresses these challenges head-on. GenerativeĀ UI offers tools that are inherently well-suited for the job at hand, reducing the friction caused by ill-fitting technology. Moreover, its user-friendly nature significantly flattens the learning curve, minimising the need for extensive training.
Ultimately this approach not only enhances adoption rates but also ensures that AI solutions integrate seamlessly into existing workflows, maximising their impact and utility for employees across various roles and skill levels.
How enterprises can make the most out of GenAI
While GenerativeĀ AI is set to transform business, history suggests that despite established firmsā advantages in talent, customers, and data, large incumbents often struggle during technological shifts.
Past technological transformations saw significant disruption, with new entrants frequently overtaking industry leaders. This isnāt due to lack of awareness ā a recent survey shows over half of executives anticipate AIās disruptive potential. The real challenge is understanding how AI can enhance current practices and make significant changes to successful business models. Newcomers, free from legacy systems, are often better at adopting new technologies.
Weāve seen this in companies like Klarna, who famously boast of over two million conversations completed by its OpenAI-powered virtual assistant. Klarna estimates that its new asset is covering the work of 700 full-time agents ā and to the same standards of service. In total, nine out of 10 Klarna staff now use GenerativeĀ AI (including the companyās in-house lawyers). The buy-now-and-pay-later company did not go about building their own AI model. Instead, they leveraged existing ones, to show-stopping effect.
But success is not limited to one company when talking about GenerativeĀ AI, and the opportunity exists for more traditional banks and financial services to streamline and transform their business processes also by combining LLM capabilities with in-house proprietary data.
The numbers are not easily excused. Some estimates suggest that the full implementation of GenerativeĀ AI in banking will deliver an additional annual value of Ā£158 billion to Ā£268 billion globally. But this will not be achieved by giving every employee a ChatGPT subscription or even launching an internal chatbot. Instead, it will be achieved by creating AI products that are designed for specific tasks and workflows that are enabled via intelligent interfaces. By developing the right AI products for the right task and correct user via GenerativeĀ UI, businesses will unlock AIās true potential.
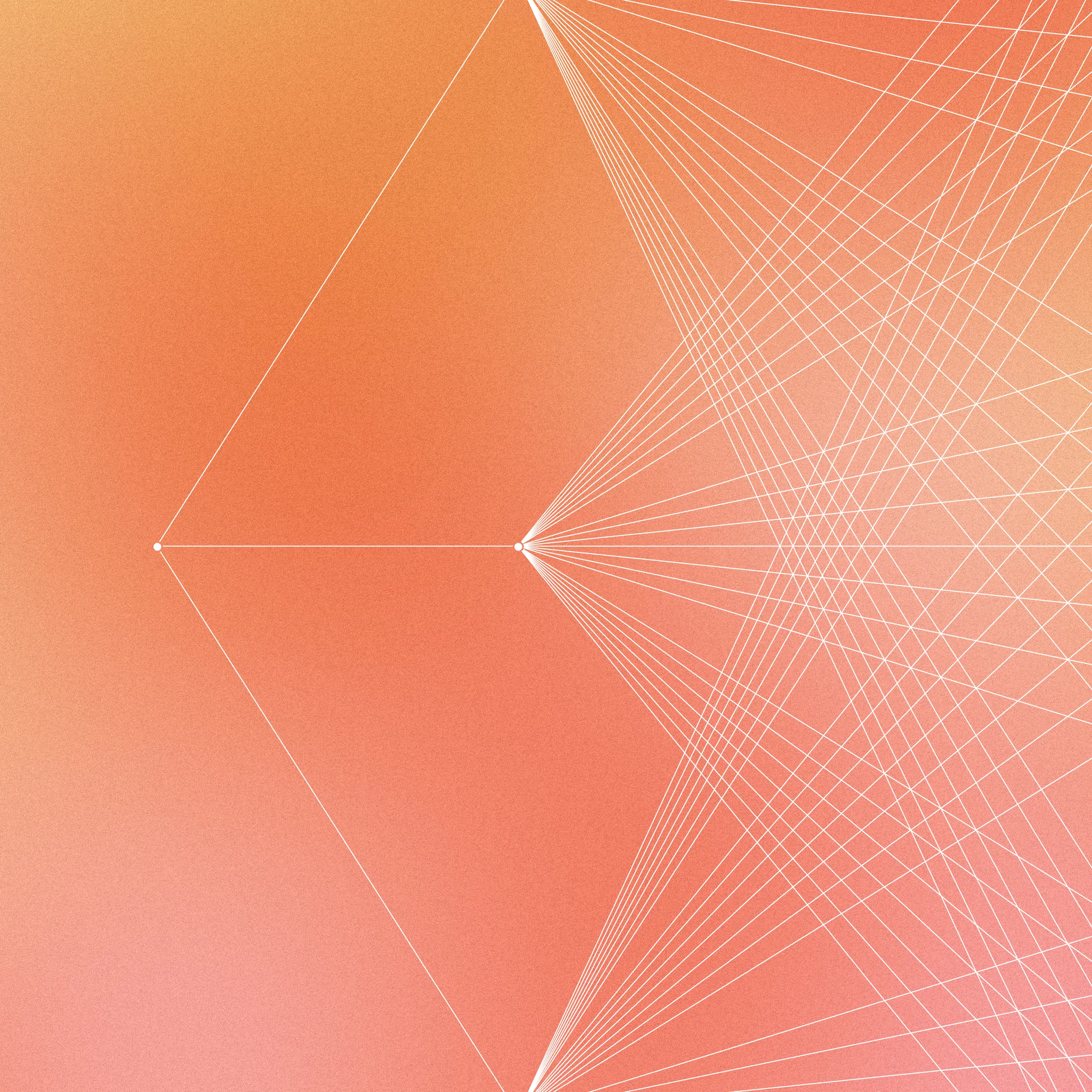
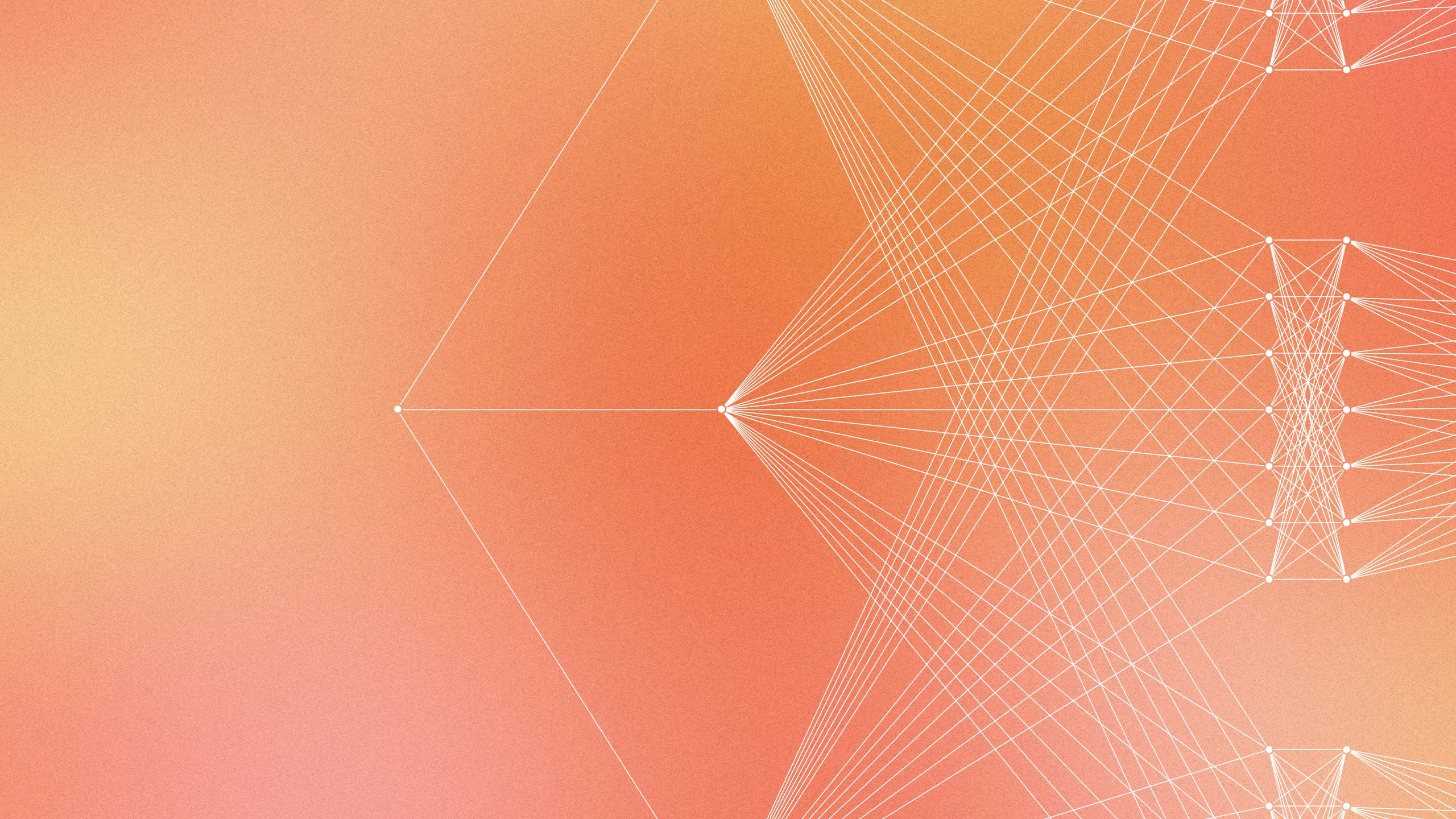
The success of ChatGPT: paving the way for GenerativeĀ UI
The success of ChatGPT and similar tools marked a pivotal moment in the evolution of AI interfaces. Their breakthrough wasnāt just in the underlying technology, but in how they presented AI to the world. For the first time, AI had a face ā or more accurately, a chat window. This simple yet powerful interface created an experience that was immediately engaging and accessible to users of all backgrounds.
By giving AI a UI, these tools effectively bridged the gap between complex language models and everyday users. They created an experience that felt both natural and revolutionary. Users could simply type their questions or requests and the AI would respond in kind, abstracting away the intricate machinery working behind the scenes.
This approach democratised access to AI capabilities that were previously the domain of specialists. It allowed people to interact with advanced language models without needing to understand the technicalities behind them. The result was a surge in adoption (upon release ChatGPT soon became the fastest spreading tech platform in history) and a fundamental shift in how people perceived and interacted with AI technology.
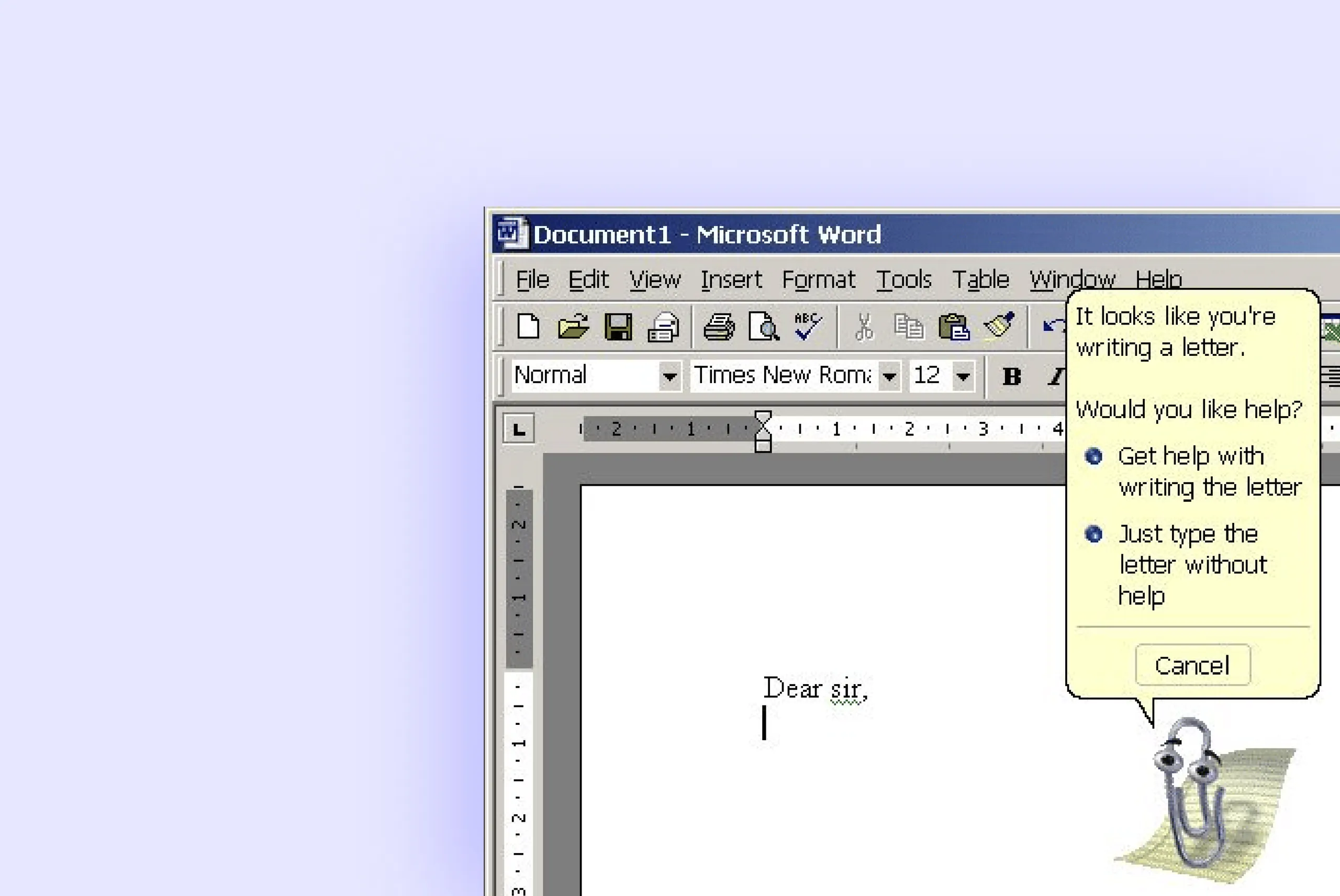
Overcoming limitations: why we need GenerativeĀ UI
Despite their groundbreaking nature, early LLM interfaces have limitations that are becoming increasingly apparent as users and businesses seek more sophisticated applications. While chatbots and text-based interfaces have made AI accessible, they often fall short in several key areas.
Firstly, these interfaces are primarily designed for general-purpose interactions, which can limit their effectiveness in specialised domains or complex workflows. Users often find themselves spending considerable time crafting precise prompts to get the desired results, a process that can be both time-consuming and frustrating.
Secondly, the reliance on text-based input and output doesnāt fully leverage the multimodal capabilities of advanced AI models. This constraint can hinder the seamless integration of AI into tasks that involve visual, auditory, or spatial elements.
Additionally, current interfaces often lack contextual awareness and struggle to maintain coherent, long-term interactions. This limitation can result in disjointed experiences, especially in scenarios requiring ongoing collaboration or project management.
Lastly, these interfaces frequently operate as standalone tools, rather than integrating smoothly with existing software ecosystems. This isolation can create friction in workflows and hinder the widespread adoption of AI in enterprise settings.
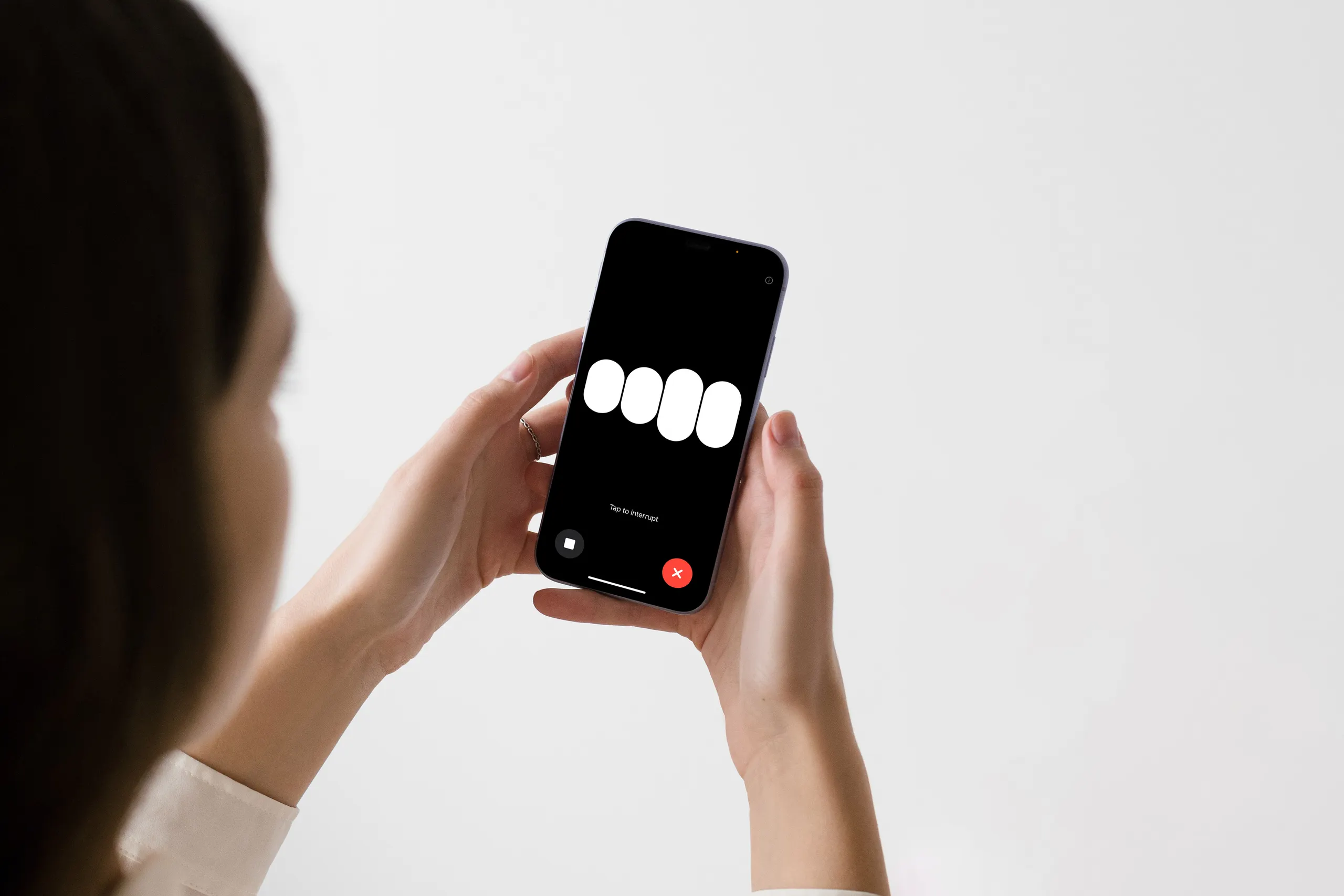
GenerativeĀ UI: creating intuitive, task-specific interfaces
While text interfaces have been revolutionary then, they also their limitations. From the offset, they often constrain our use of LLMs and other AI tools to patterns weāre already familiar with from existing digital tools.
Recent developments are pushing beyond these constraints. OpenAIās GPT4-o and Googleās Project Astra represent significant advancements towards systems that work intuitively with their context. These systems accept multimodal input ā not just text, but also vision and audio. This multimodal approach allows AI to perceive and interact with the world in a more human-like manner, greatly enhancing their utility and accessibility.
This shift towards multimodal, task-specific interfaces is crucial. It allows us to move beyond the constraints of familiar digital interaction patterns and create AI tools that truly augment human capabilities.
For instance, a video editing AI assistant might offer a timeline-based interface rather than a chat window, aligning with editorsā existing mental models and workflows. Similarly, an AI-powered architectural design tool could present a 3D modelling environment, allowing architects to manipulate structures using familiar gestures and commands. For medical professionals, an AI diagnostic aid could integrate seamlessly with existing imaging software, overlaying insights directly onto scans and patient records.
These task-specific interfaces abstract away the complexity of AI interactions, presenting users with familiar tools and metaphors relevant to their specific needs. In this sense, intelligent interfaces allow users to focus on the task at hand, not the technology underpinning it. This is where the true value lies ā in how well the tool solves the particular userās job (JTBD) and how effectively you can improve it by leveraging different models.
With GenerativeĀ AI, intelligence itself is becoming a commodity, the key differentiator is about shaping that commodity to solve the right problem in the right way.
The future of AI is less Microsoft Clippy, and more Apple intelligence.
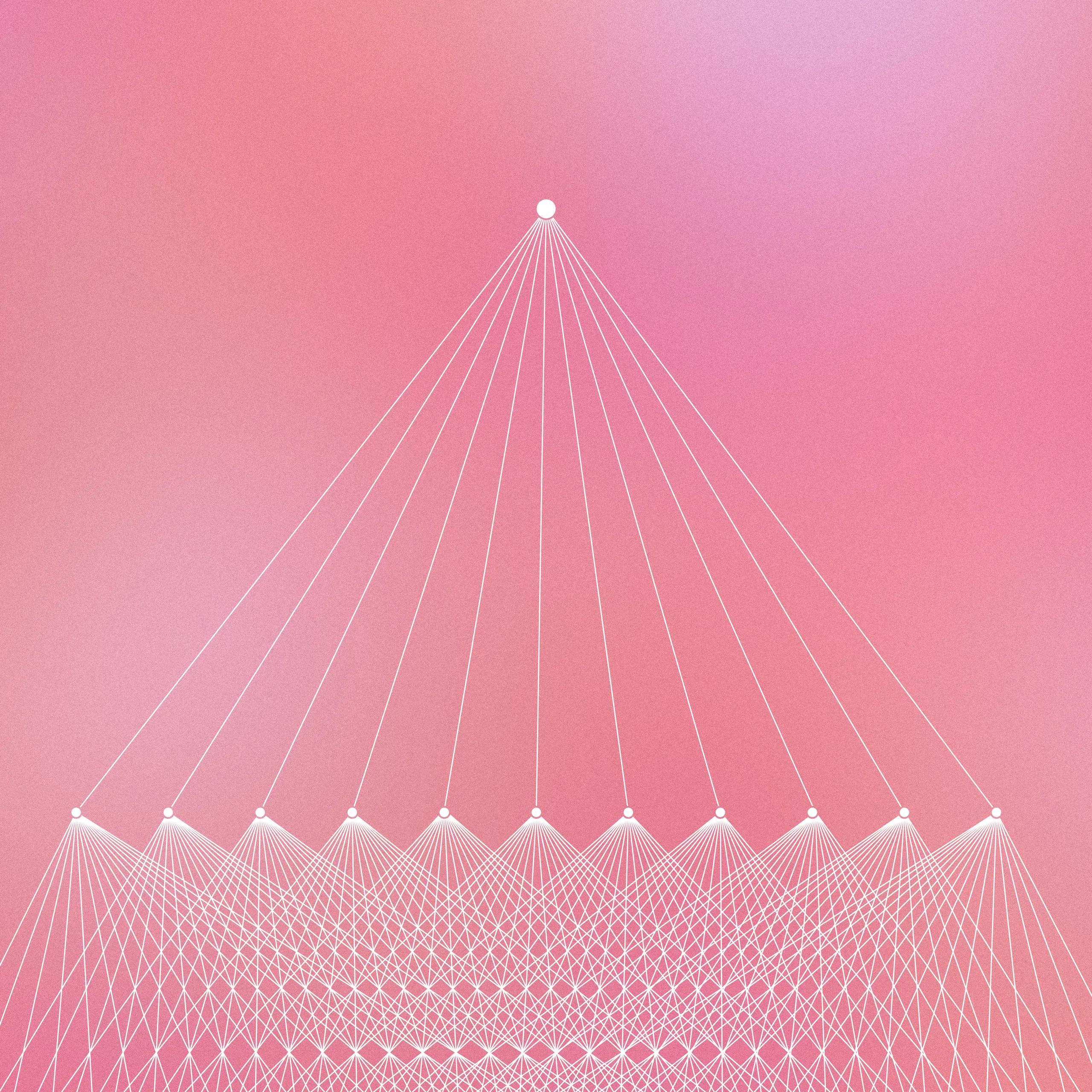
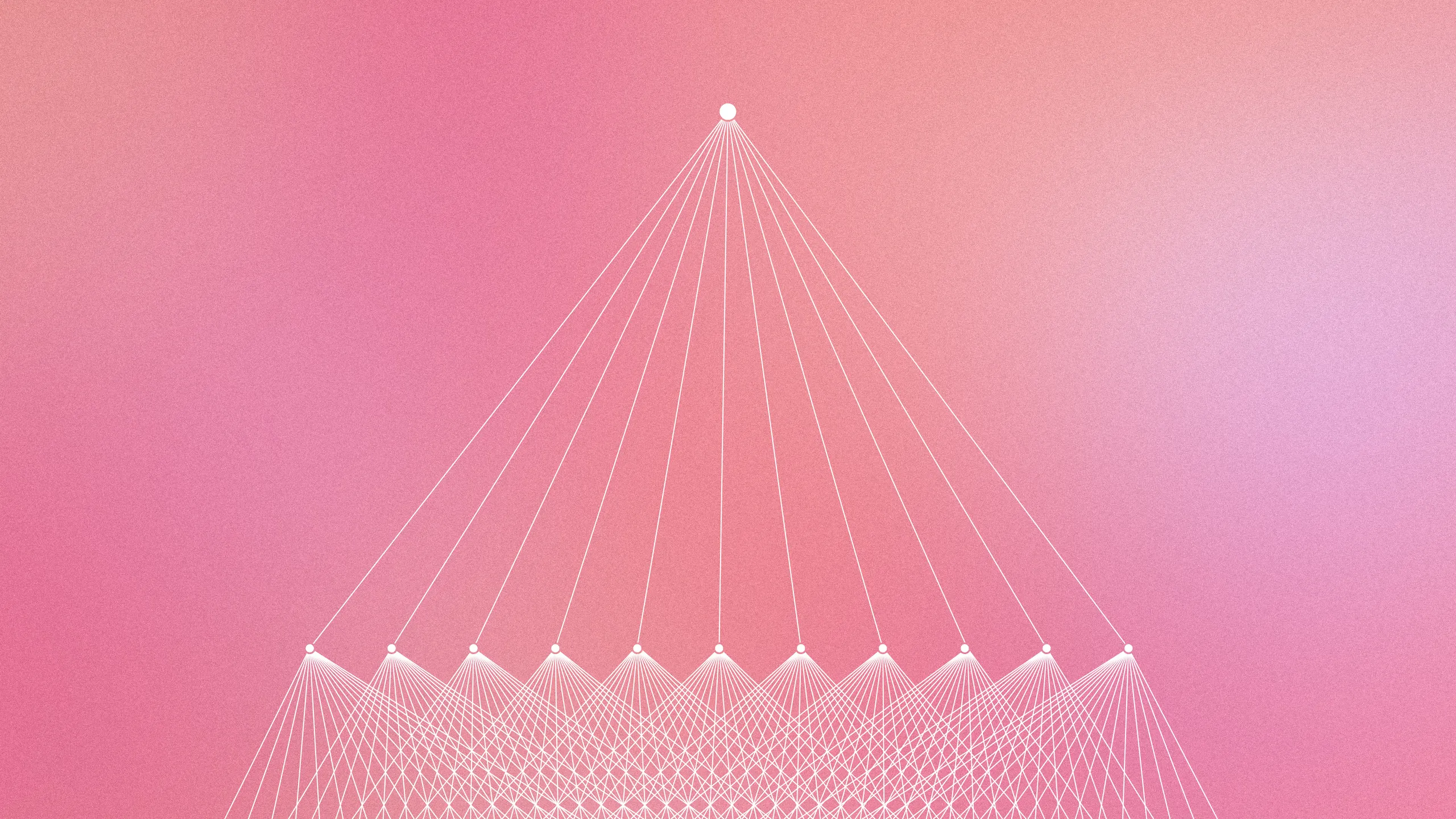
GenerativeĀ UI: shaping the future of AI Interfaces
The conversation around GenerativeĀ AI has been dominated by discussions of foundational infrastructure, particularly LLMs, for the past two years. While this focus has been crucial for establishing the groundwork, itās time to shift our attention towards developing AI applications that address real-world problems and create tangible value.
As we move forward, the emphasis will be on launching AI-native products, services, and businesses that have the potential to disrupt existing market paradigms. This shift represents a maturation of the AI landscape, where the technology moves from being a subject of theoretical discourse to a practical and accessible tool for innovation and problem-solving.
The next generation of AI interfaces will play a crucial role in this transition. These interfaces will prioritise the user experience by abstracting the complexities of AI systems behind intuitive designs. Instead of requiring users to craft precise prompts or understand the intricacies of underlying AI models, these interfaces will present familiar, task-oriented tools that seamlessly integrate AI capabilities.
Transition from chatbots to adaptive interfaces
The transition moves us away from complex AI interfaces towards intuitive, user-friendly experiences that donāt require specialised knowledge or skills. By eliminating the need for users to craft precise prompts or understand
AI jargon, weāre democratising access to powerful AI capabilities.
This focus on accessibility and seamless interaction is crucial for driving widespread AI adoption across different sectors and demographics. It ensures that the benefits of AI are not limited to tech-savvy users but are available to anyone who can benefit from these powerful tools, leading to greater and wider impact.
Take Apple Intelligence for instance, which exemplifies this philosophy by prioritising user experience. Rather than overtly showcasing AI capabilities, Apple integrates them subtly into existing interfaces and workflows. Contrast this with Microsoftās more visible approach seen in tools like Co-Pilot, which requires users to learn new interaction paradigms and essentially slaps a chat interface atop of existing tools.
Domain-specific modelling and its benefits
Forward-thinking companies are now integrating Large Language Models (LLMs) into their proprietary applications and workflows, harnessing domain-specific data to create more targeted and effective AI that sits behind intelligent interfaces.
This integration marks a shift from generic AI solutions to more tailored, domain- specific implementations. As businesses seek to leverage AIās full potential within their unique contexts, theyāre exploring various strategies to adapt LLMs to their specific needs.
There are three main approaches to implementing domain-specific LLMs: building from scratch, fine-tuning pre-trained models, and enhancing prompts with relevant data.
Building from scratch offers the highest degree of customisation but is also the most resource-intensive option. It requires substantial data science expertise, large volumes of high-quality data, and significant computational power ā the result of which is that many businesses simply canāt do it due to huge cost.
Instead, the trend is moving towards more efficient and cost-effective approaches that leverage existing models and technologies: Fine- tuning and Retrieval Augmented Generation (RAG).
Whilst fine-tuning has received a lot of attention over the past two years, focusing on fundamentals like data quality, effective prompting, and RAG has often been found to yield better results. A paper from January 2024 assessed the effectiveness of different LLM enhancement techniques using the Massively Multilingual Language Understanding Evaluation (MMLU) benchmark. The study compared RAG, fine-tuning, and a combination of both approaches.
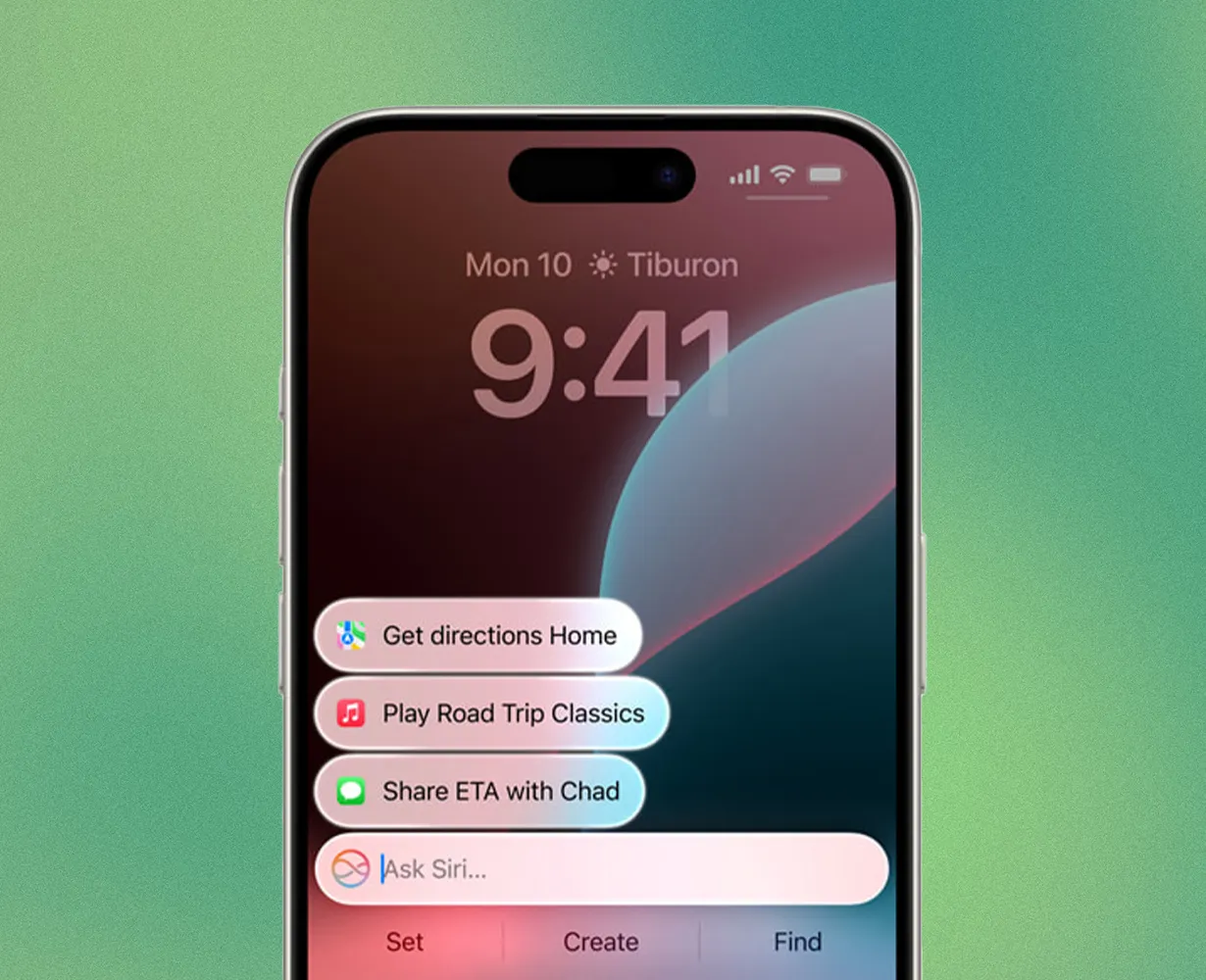
RAG consistently improved the base modelās accuracy, showing gains in all tested domains. Fine-tuning also generally outperformed the base model, but RAG proved more effective in most scenarios. Specifically, fine-tuning only surpassed RAG in two cases, and by a narrow margin.
The researchers also explored combining fine-tuning with RAG. This hybrid approach sometimes outperformed RAG alone, but RAG still maintained superior performance in approximately 75% of cases. At Elsewhen, weāve recognised the power and versatility of RAG, and itās become our go-to approach for many client projects.
By providing the model with trusted, domain-specific information as part of the prompt, RAG reduces the risk of inaccuracies or āhallucinationsā common in general-purpose models. This method doesnāt require the extensive data science expertise of building from scratch or fine-tuning, but it does necessitate robust data management practices to ensure the right information is being fed into the model.
The cutting-edge nature of GenerativeĀ AI of course means that everyday, new techniques are developing. Model merging is such a technique, and allows for the combination of multiple models by merging them into one.
Another development is the application of Small Language Models (SLMs), AI models designed to provide powerful performance in compact form. SLMs are set to offer accurate results at a much lower cost for businesses, trained on more precise and controlled data sets which improve accuracy.
At the end of the day, it is likely that for many AI applications, wrangling information from massive maelstroms of data is not the best approach. In the near future, smaller, more tailored models will be produced that are dedicated to the task at hand (after all, not every solution also needs to know the philosophies of Ancient Greece).
In any case, such developments reinforce the need for any AI solution to be built with interoperability in mind. Flexible in both the user interface and in the underlying models and technology.
The rise of GenerativeĀ UI: beyond traditional AI Interfaces
The concept of generating the right UI at the right moment goes beyond just adapting existing interfaces. GenerativeĀ UI represents a fundamental shift in how we create and interact with user interfaces. At its core, it leverages AI modelsā ability to generate code in real-time, enabling the creation of entirely new UI elements and interactions on demand.
What this all ladders up towards is a world wherein everything from sound to gestures, text to images, becomes not just an input, but a catalyst for generating tailored interface components. The AI doesnāt simply respond to these inputs; it uses them to craft bespoke UI elements by writing new code that best serve the userās current needs.
By combining various types of foundational models, LLMs can act as a sophisticated, intelligent UI generator. In doing so, they can analyse user inputs, context, and historical data to not only invoke specific AI models as needed but also render the UI in real-time in response to the AI systemās needs ā transforming the interface from a static, pre-designed construct into a fluid, code-generating entity.
This approach revolutionises how we think about UI/UX design, building upon and dramatically extending existing paradigms like design systems and atomic design principles. By leveraging the modularity and consistency of design systems, GenerativeĀ UI takes these concepts to their logical conclusion: a living, breathing interface that reshapes itself to perfectly meet the needs of every user in every moment across every context ā not just by adapting existing elements, but by creating new ones as needed.
Enhancing UX and productivity: the GenerativeĀ UI impact
This more intuitive interaction with machines will inevitably lead to productivity gains as they will require less training to use effectively. In doing so, it will remodel every job as an AI-enabled one.
A recent study from Denmark, which surveyed 100,000 workers across 11 occupations, for example, revealed significant challenges in the adoption of AI tools like ChatGPT. The study found that 43% reported needing training to use it effectively ā a barrier particularly affecting women, with 48% citing this issue compared to 37% of men. Likewise, 35% of workers faced employer restrictions on ChatGPT use.
Despite these challenges, workers recognised the potential of AI, estimating that ChatGPT could halve working times in about 37% of their job tasks.
Intelligent, adaptive, task-specific interfaces can solve such hurdles by addressing employer concerns, reducing training needs, bridging the gender gap in AI adoption, and helping realise the significant productivity potential of AI tools.
Democratising data access with GenerativeĀ UI
By providing a user-friendly platform for all data users, from novices to experts, GenerativeĀ UI can significantly enhance data accessibility and utilisation across organisations. Gartnerās prediction that over 80% of enterprises will deploy GenAI applications by 2026, up from less than 5% in 2023, underscores this technologyās explosive growth and potential.
Data democratisation means enhanced efficiency by streamlining repetitive tasks like email writing, coding, and document summarisation, freeing up valuable time for more strategic work. GenAI can also boost overall business productivity by enabling more efficient approaches to high-value tasks. Furthermore, personalised AI assistants can help employees quickly become proficient in new tasks, fostering a more adaptable workforce.
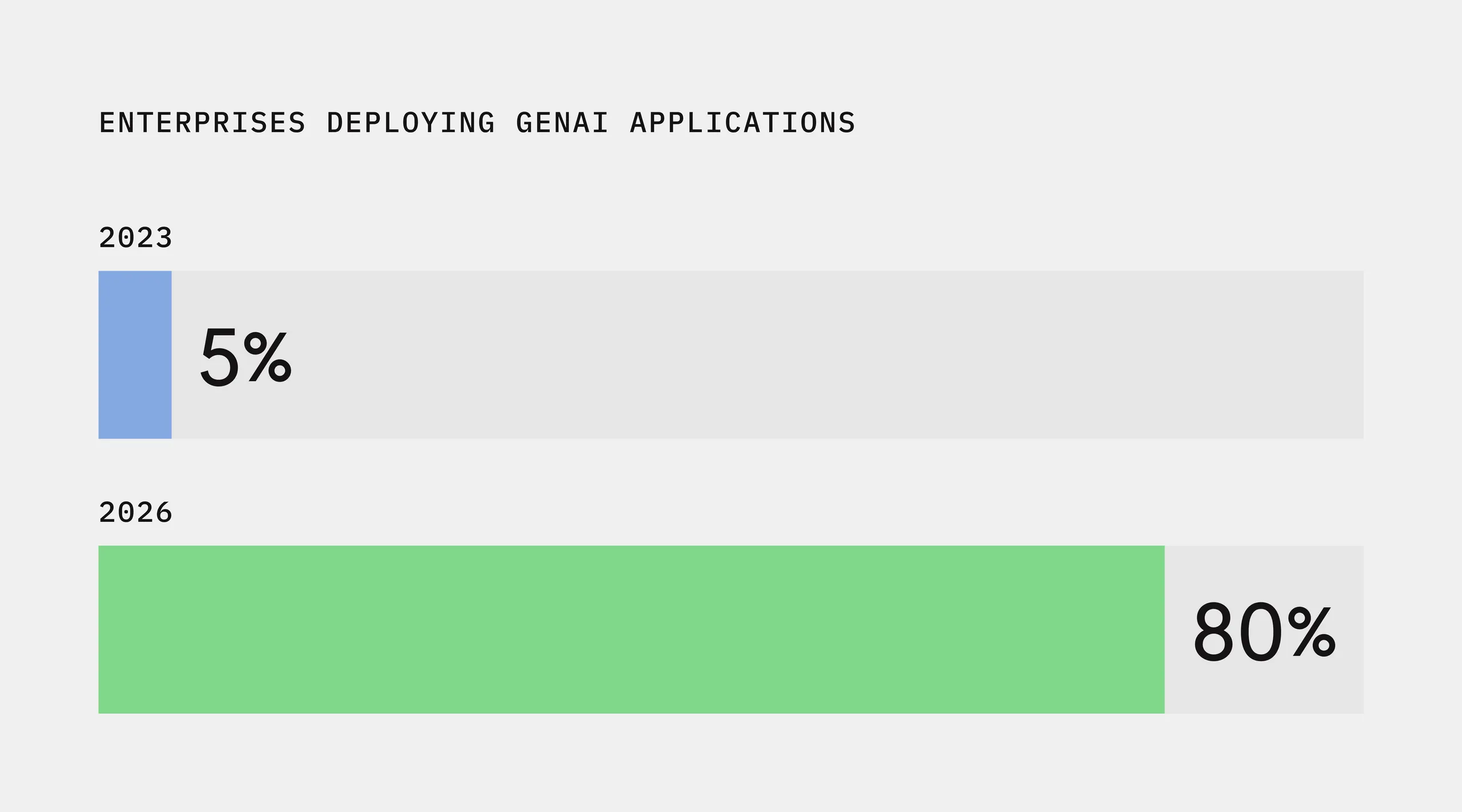
In August 2024, the bank JPMorgan announced LLM Suite ā a GenerativeĀ AI tool for its workers ā and stated that it was already helping more than 60,000 employees with tasks like writing emails and reports. As with many enterprises, rather than developing its own AI models, JPMorgan designed LLM Suite to be a portal that allows users to tap external LLMs.
Elsewhere, the fashion retailer Mango, which recorded record growth in 2023, and a record first half of 2024, boasts 15 separate AI tools across the enterprise. One of these tools is Lisa, a conversational generativeĀ AI platform that aids employees with various tasks including trend analysis, designing its collections and supporting customer service processes.
This is where one of the greatest opportunities of GenAI lies: in revolutionising information access by making finding and retrieving information easier through contextual search and conversational interfaces. This democratisation of knowledge across organisations has the potential to level the playing field and spark innovation at all levels, creating multiple boons across the organisation. In doing so, itās liberating data from organisational silos and unstructured formats like documents, where valuable insights often remain hidden.
GenAI models can now intelligently parse through vast amounts of unstructured data, extracting relevant information and presenting it in structured, accessible ways.
This capability allows for the right data to be delivered at the right time, tailored to the specific needs of each user. As a result, weāre seeing a new level of vibrancy and fluidity in organisational data flow. Employees across all levels can now access previously hard-to-reach information, making them better informed and more capable of making improved actions and decisions.
As intelligent interfaces continue to democratise knowledge across the enterprise, their impact on business operations and innovation will be profound.
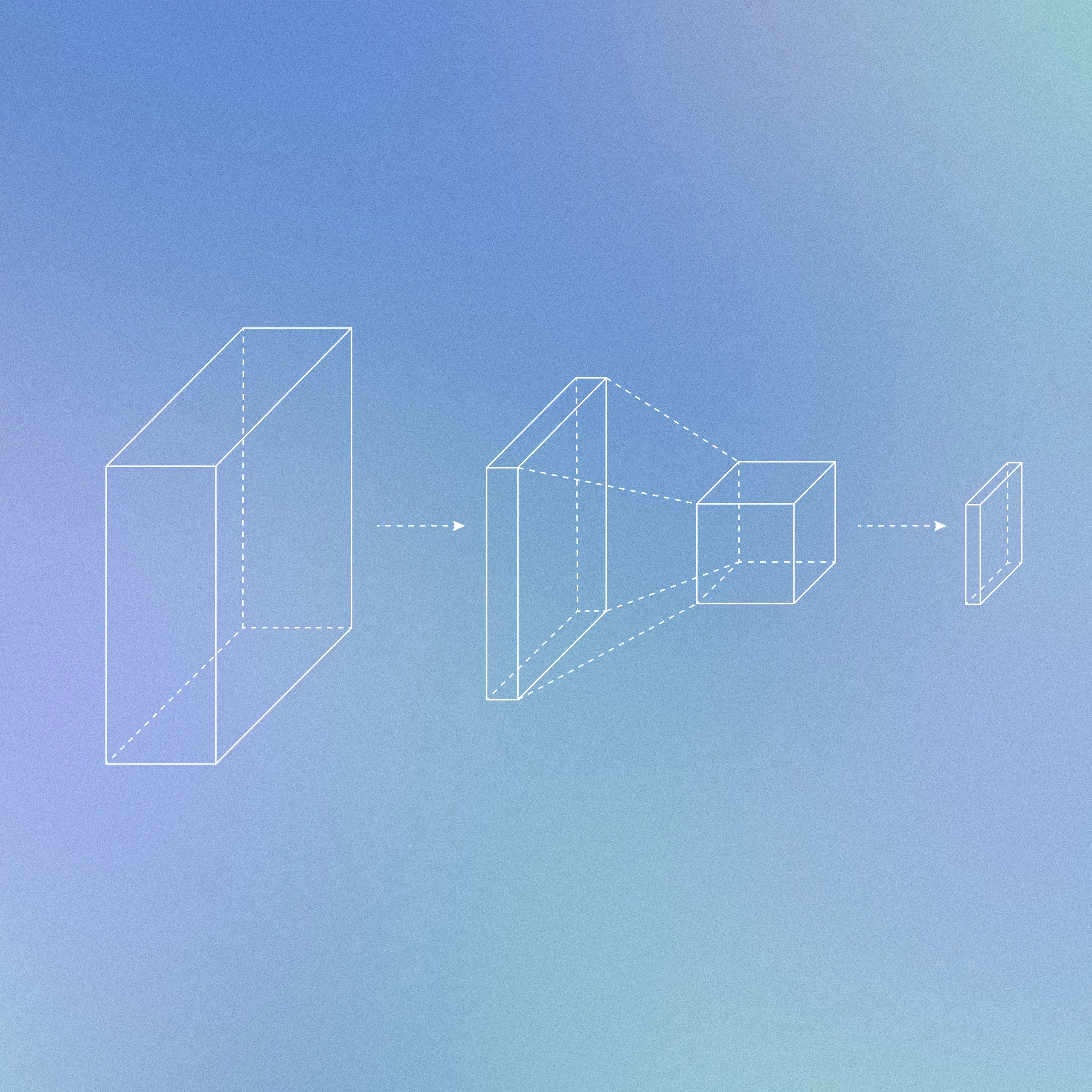
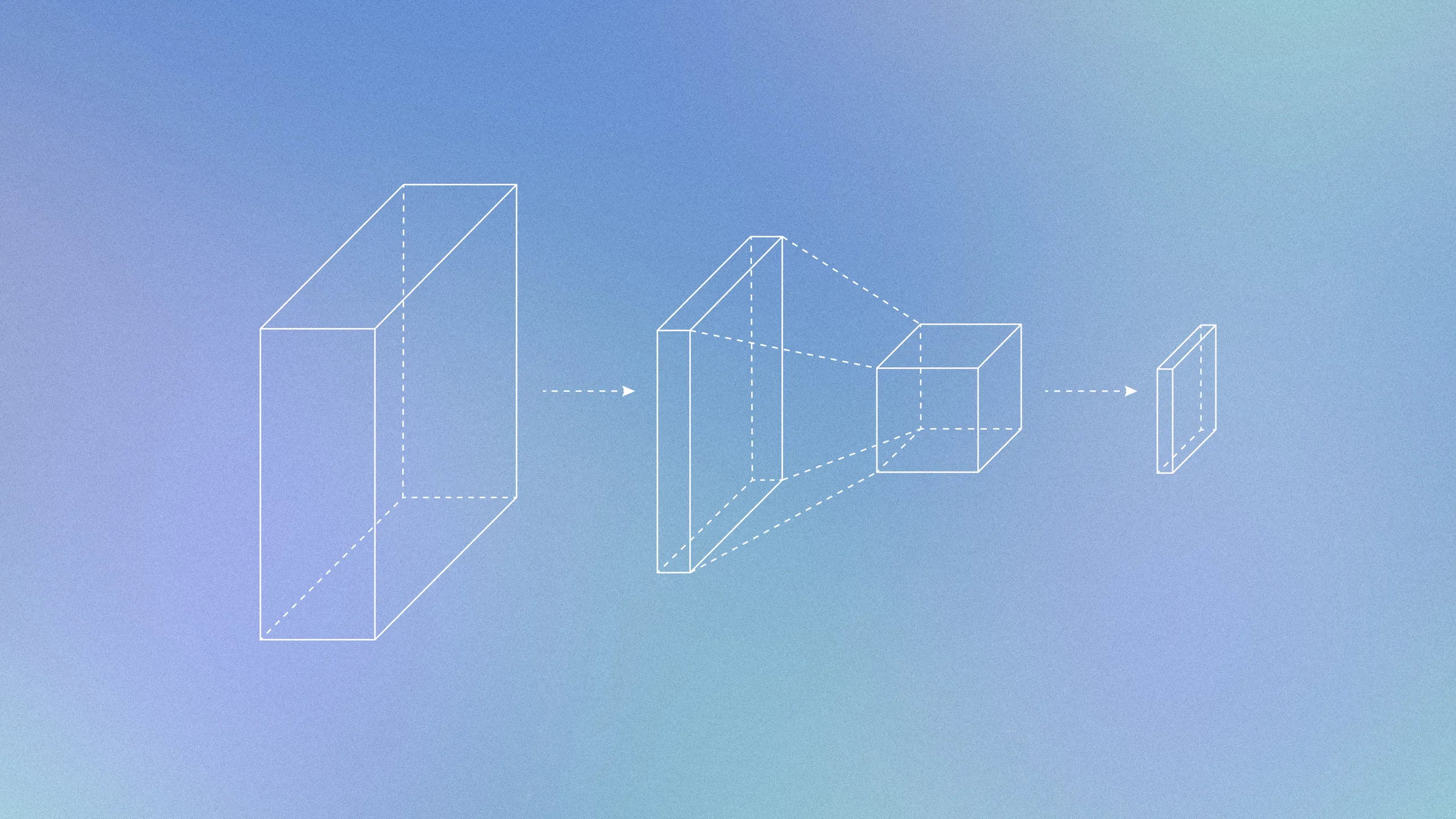
The GenerativeĀ UI opportunity: GenerativeĀ UI represents a leap beyond current GenerativeĀ AI tools, redefining the landscape of human-machine interaction.
While chatbotsā success catalysed widespread adoption and acceptance of AI in everyday interactions, they were only the first step towards a future where AI seamlessly integrates into every facet of our digital experiences.
GenerativeĀ UI is the next stage in this evolution. By seamlessly managing multimodal interactions, leveraging diverse AI models, and applying domain-specific knowledge, it presents users with an interface so intuitive it borders on the magical.
The enhanced productivity will create an environment ripe for innovation and experimentation. On an individual level, GenerativeĀ UI will allow users to fail faster and build better. At an enterprise level, adoption hurdles will dissipate as tools that adapt to the user and task at hand. Once again, itās not about farming everything out to the machine. Instead, it is about augmenting us to do more with less.
As we move from chatbots to more sophisticated, multimodal GenerativeĀ AI systems, the future will be one defined by GenerativeĀ UI: interfaces that dynamically adapt to user needs, abstracting complex AI behind seamless experiences.